In today’s data-driven and technologically advanced business landscape, the strategic integration of Artificial Intelligence (AI) has become a pivotal decision for organizations seeking to gain a competitive edge.
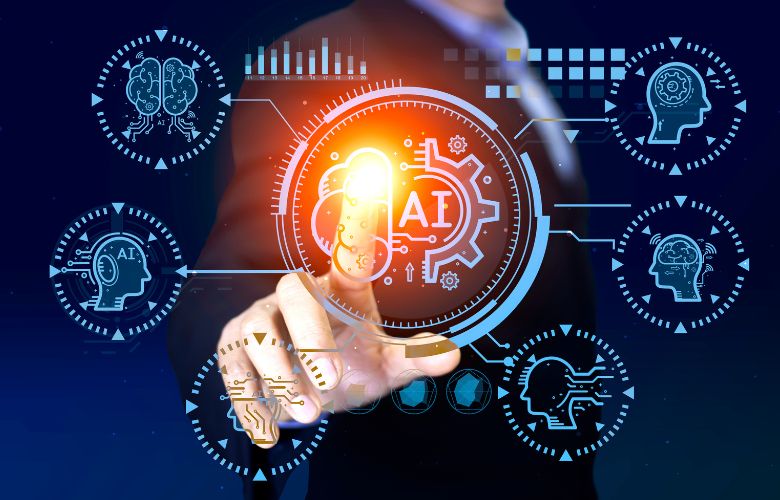
Contents
Introduction
Artificial Intelligence has revolutionized industries by enhancing operational efficiency, customer experiences, and decision-making processes. To identify the business cases where AI shines the brightest, we will explore five key scenarios. Each case will be assessed based on its potential impact, data requirements, and the AI techniques involved.
Business Cases for AI Implementation
2.1 Customer Relationship Management (CRM)
Problem Statement: Enhancing customer relationships and driving sales growth are perennial challenges for businesses. AI can be employed to analyze vast customer data, predict purchase behavior, and personalize interactions.
Data Requirements: Customer data, purchase history, social media interactions, and demographic information.
AI Techniques: Machine learning, natural language processing, and predictive analytics.
2.2 Predictive Maintenance
Problem Statement: Equipment breakdowns and unplanned downtime can be costly. Predictive maintenance powered by AI can forecast when machinery needs repair, reducing operational disruptions.
Data Requirements: Historical equipment data, sensor readings, maintenance logs, and environmental factors.
AI Techniques: Time-series analysis, anomaly detection, and machine learning algorithms.
2.3 Financial Fraud Detection
Problem Statement: Financial institutions face constant threats from fraudsters. AI can analyze transaction data in real-time, identifying suspicious activities and potential fraud.
Data Requirements: Transaction records, customer profiles, historical fraud data, and behavioral patterns.
AI Techniques: Anomaly detection, machine learning, and pattern recognition.
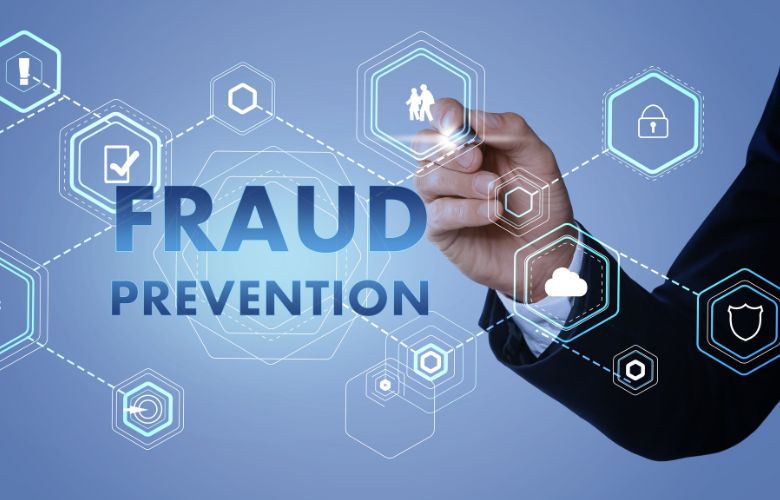
2.4 Supply Chain Optimization
Problem Statement: Efficient supply chain management is critical for cost reduction. AI can optimize inventory levels, demand forecasting, and route planning.
Data Requirements: Inventory data, historical sales data, supplier data, and transportation data.
AI Techniques: Demand forecasting models, optimization algorithms, and machine learning.
2.5 Personalized Marketing
Problem Statement: Generic marketing strategies often yield subpar results. AI can analyze customer preferences and behavior to deliver tailored marketing campaigns.
Data Requirements: Customer behavior data, purchase history, website interactions, and demographic data.
AI Techniques: Customer segmentation, recommendation engines, and natural language processing.
Data Table: A Comparative Overview
Let’s compare these business cases based on specific parameters:
Parameter | Customer Relationship Management | Predictive Maintenance | Financial Fraud Detection | Supply Chain Optimization | Personalized Marketing |
Potential Impact | Enhanced customer satisfaction, increased sales | Reduced downtime, cost savings | Fraud prevention, cost savings | Improved efficiency, cost savings | Increased conversion rates, revenue |
Data Requirements | Customer data, purchase history, social interactions, demographics | Historical equipment data, sensor readings, maintenance logs | Transaction records, customer profiles, historical fraud data | Inventory data, sales history, supplier data, transportation data | Customer behavior data, purchase history, website interactions, demographics |
AI Techniques | Machine learning, natural language processing, predictive analytics | Time-series analysis, anomaly detection, machine learning | Anomaly detection, machine learning, pattern recognition | Demand forecasting models, optimization algorithms, machine learning | Customer segmentation, recommendation engines, natural language processing |
Key Takeaways
- Tailored Solutions: AI is not a one-size-fits-all solution. It thrives in scenarios where data-driven insights can be harnessed to deliver personalized solutions.
- Impact Metrics: Consider the specific metrics for measuring success in each case. Whether it’s increased revenue, cost savings, or customer satisfaction, align AI implementation with desired outcomes.
- Data Accessibility: Assess your organization’s data infrastructure. Availability and quality of data play a crucial role in the successful deployment of AI.
- AI Expertise: The choice of AI techniques and models requires expertise. Organizations may need to invest in AI talent or collaborate with AI service providers.
- Continuous Improvement: AI is an evolving field. Continuous monitoring and refinement of AI models are essential for sustained success.
Conclusion
In the pursuit of leveraging Artificial Intelligence to drive business growth and efficiency, it’s crucial to select the right business case. The choice should align with organizational goals, data availability, and the potential for AI-driven impact.
Each of the business cases we explored—Customer Relationship Management, Predictive Maintenance, Financial Fraud Detection, Supply Chain Optimization, and Personalized Marketing—offers unique opportunities for AI implementation. The key lies in identifying which case aligns most closely with your business objectives and data capabilities.
Remember that AI is not a silver bullet but a tool that, when applied strategically, can unlock transformative results. To make an informed decision, assess your organization’s readiness, objectives, and the potential impact of AI implementation. Armed with these insights, you can embark on a journey to harness the power of Artificial Intelligence and drive your business towards a brighter, data-driven future.